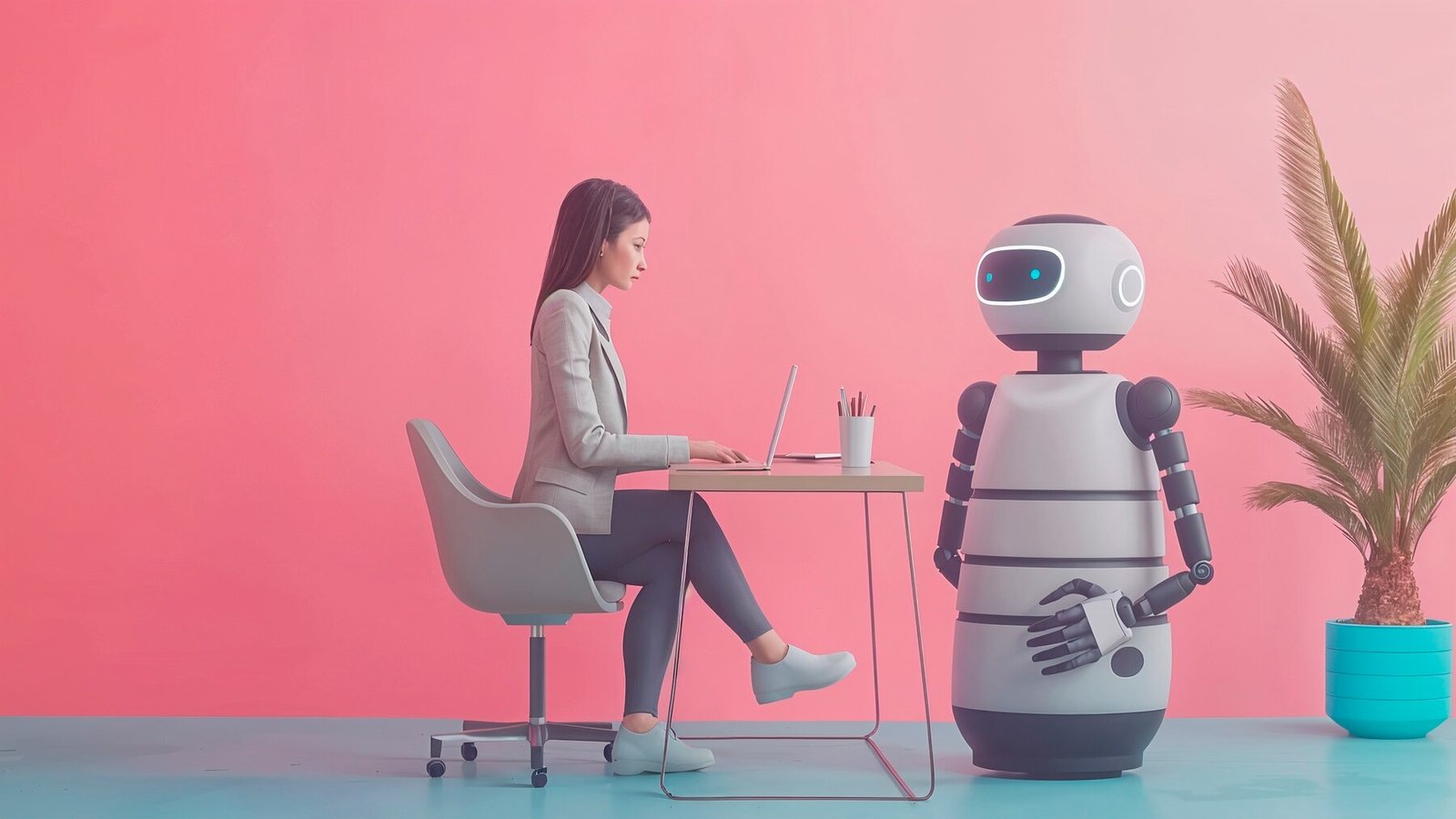
Artificial Intelligence (AI) has the potential to revolutionize industries, streamline operations, and drive innovation. However, the path to successful AI implementation is fraught with challenges. From technical hurdles to ethical considerations, organizations face numerous obstacles when integrating AI into their operations. This blog explores the key challenges of AI implementation, the lessons learned from various projects, and strategies for overcoming these difficulties.
1. Data Quality and Availability
Challenge: AI systems rely heavily on data for training and decision-making. Poor quality data or insufficient data can lead to inaccurate predictions and unreliable results. Data may be incomplete, outdated, or biased, which can compromise the effectiveness of AI models.
Lesson Learned: Ensuring data quality and availability is critical. Organizations should invest in robust data governance practices, including data cleansing, validation, and integration. Establishing clear data management policies and leveraging advanced data analytics tools can help improve data quality and ensure that AI models are trained on reliable information.
Strategy: Implement data management frameworks and invest in data preprocessing tools. Regularly audit data sources and employ techniques such as data augmentation to enhance the quality and quantity of data available for AI training.
2. Integration with Existing Systems
Challenge: Integrating AI solutions with existing systems and workflows can be complex and disruptive. Legacy systems may not be compatible with new AI technologies, leading to integration challenges and operational disruptions.
Lesson Learned: Successful AI implementation requires careful planning and a phased approach. Organizations should conduct thorough assessments of their existing infrastructure and identify integration points before deploying AI solutions. Collaborative efforts between IT and business units are essential to ensure seamless integration and minimize disruptions.
Strategy: Develop a clear integration plan that outlines the technical requirements and potential challenges. Consider adopting modular AI solutions that can be incrementally integrated with existing systems to reduce the risk of operational disruptions.
3. Skills and Expertise
Challenge: The successful implementation of AI requires specialized skills and expertise, including data science, machine learning, and software engineering. A shortage of skilled professionals can hinder AI adoption and limit the effectiveness of AI initiatives.
Lesson Learned: Building a skilled AI team is crucial for successful implementation. Organizations should invest in training and upskilling programs for existing employees and consider partnering with external experts or consultants to fill skill gaps.
Strategy: Develop a talent acquisition and development strategy that includes recruiting AI professionals, offering training programs, and fostering a culture of continuous learning. Explore partnerships with educational institutions and industry experts to stay updated with the latest advancements in AI.
4. Ethical and Bias Concerns
Challenge: AI systems can perpetuate and amplify biases present in the training data, leading to ethical concerns and potential discrimination. Ensuring fairness and transparency in AI decision-making is a significant challenge.
Lesson Learned: Addressing ethical concerns and bias requires a proactive approach. Organizations should implement fairness and transparency measures, including bias detection and mitigation techniques. Engaging diverse teams in the development process and conducting regular audits can help identify and address ethical issues.
Strategy: Establish ethical guidelines for AI development and deployment. Implement tools and practices for bias detection and correction, and involve diverse stakeholders in the AI development process to ensure that the technology is fair and equitable.
5. Privacy and Security
Challenge: AI systems often handle sensitive data, raising concerns about privacy and security. Data breaches or unauthorized access to AI systems can lead to significant risks, including loss of confidential information and regulatory non-compliance.
Lesson Learned: Implementing strong security measures and adhering to privacy regulations are essential for safeguarding data and maintaining trust. Organizations should prioritize data protection and conduct regular security assessments to identify and address potential vulnerabilities.
Strategy: Develop and enforce robust data security policies and practices, including encryption, access controls, and regular security audits. Ensure compliance with relevant data protection regulations and standards, such as GDPR or CCPA.
6. Change Management
Challenge: AI implementation often requires changes in organizational processes, workflows, and employee roles. Resistance to change and lack of buy-in from stakeholders can impede the successful adoption of AI technologies.
Lesson Learned: Effective change management is crucial for AI adoption. Organizations should communicate the benefits of AI clearly, involve stakeholders in the implementation process, and provide training and support to ease the transition.
Strategy: Develop a comprehensive change management plan that includes communication strategies, stakeholder engagement, and training programs. Address concerns and feedback from employees and ensure that they understand the value and impact of AI technologies.
7. Measuring and Evaluating Impact
Challenge: Assessing the impact of AI initiatives can be challenging, particularly in terms of quantifying benefits and ROI. Organizations may struggle to measure the effectiveness of AI systems and determine their contribution to business objectives.
Lesson Learned: Establishing clear metrics and evaluation criteria is essential for measuring the success of AI initiatives. Organizations should define specific goals and KPIs, and regularly assess the performance and impact of AI systems against these benchmarks.
Strategy: Develop a framework for measuring and evaluating the impact of AI initiatives, including setting clear objectives, defining relevant metrics, and conducting regular performance reviews. Use data-driven insights to refine and optimize AI systems over time.